Pre-Processing
The CSV input file used in this example:
Download CSV:
data_basic-pre-processing.csv
Surface Area,Rooms,Swimming Pool,Location,Price 300,12,yes,village,542000 134,10,no,city,320000 76,4,no,city,133000 245,7,,countryside,356000 31,2,no,city,81000 78,3,no,village,98000 123,,yes,countryside,296000 187,9,no,city,405000 ,5,yes,village,189000 367,15,yes,countryside,415000
Installing sklearn and pandas:
conda install -c anaconda scikit-learn
conda install -c conda-forge pandas
Code:
import pandas as pd
import numpy as np
from sklearn.impute import SimpleImputer
from sklearn.preprocessing import (LabelEncoder, OneHotEncoder, )
from sklearn.compose import ColumnTransformer
from sklearn.model_selection import train_test_split
from sklearn.preprocessing import StandardScaler
# Importing dataset
dataset = pd.read_csv('data_basic-pre-processing.csv')
X = dataset.iloc[:, :-1].values
y = dataset.iloc[:, -1].values
y = y.reshape((len(y), 1))
# Taking care of missing data
# Replacing missing data by mean value
imputer = SimpleImputer(missing_values=np.nan, strategy='mean')
imputer = imputer.fit(X[:, 0:1])
X[:, 0:1] = imputer.transform(X[:, 0:1])
# Replacing missing data by median value
imputer = SimpleImputer(missing_values=np.nan, strategy='median')
imputer = imputer.fit(X[:, 1:2])
X[:, 1:2] = imputer.transform(X[:, 1:2])
# Replacing missing data by most frequent value
imputer = SimpleImputer(missing_values=np.nan, strategy='most_frequent')
imputer = imputer.fit(X[:, 2:3])
X[:, 2:3] = imputer.transform(X[:, 2:3])
# Encoding categorical data
labelencoder_X = LabelEncoder()
X[:, 3] = labelencoder_X.fit_transform(X[:, 3])
X[:, 2] = labelencoder_X.fit_transform(X[:, 2])
ct = ColumnTransformer(
[("hotencoder", OneHotEncoder(categories='auto'), [3]), ],
remainder="passthrough"
)
X = ct.fit_transform(X)
# Avoiding the dummy variable trap
X = X[:, 1:]
# Splitting the dataset into the training set and test set
X_train, X_test, y_train, y_test = train_test_split(X, y, test_size=0.2)
# Features scaling
# Standarization: sklearn.preprocessing.StandardScaler()
# X' = (X - mean) / variance
# Normalization: sklearn.preprocessing.MinMaxScaler()
# X' = (X - Xmin) / (Xmax - Xmin) [0 - 1]
sc_X = StandardScaler()
X_train = sc_X.fit_transform(X_train)
X_test = sc_X.transform(X_test)
sc_y = StandardScaler()
y_train = sc_y.fit_transform(y_train)
y_test = sc_y.transform(y_test)
Input original data:
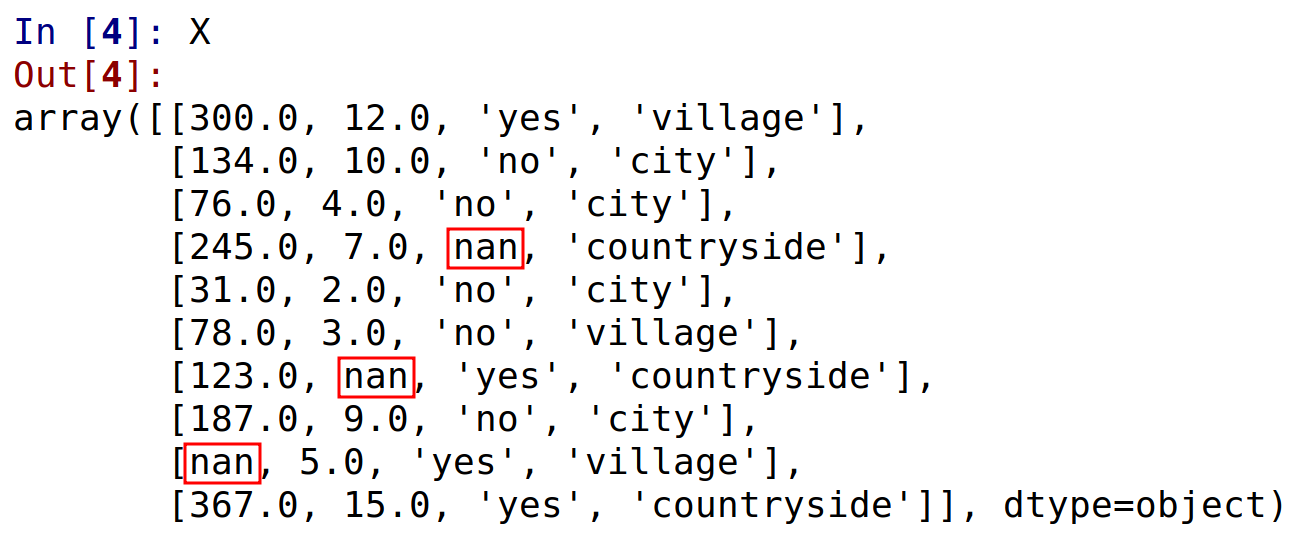
After filling missing data:
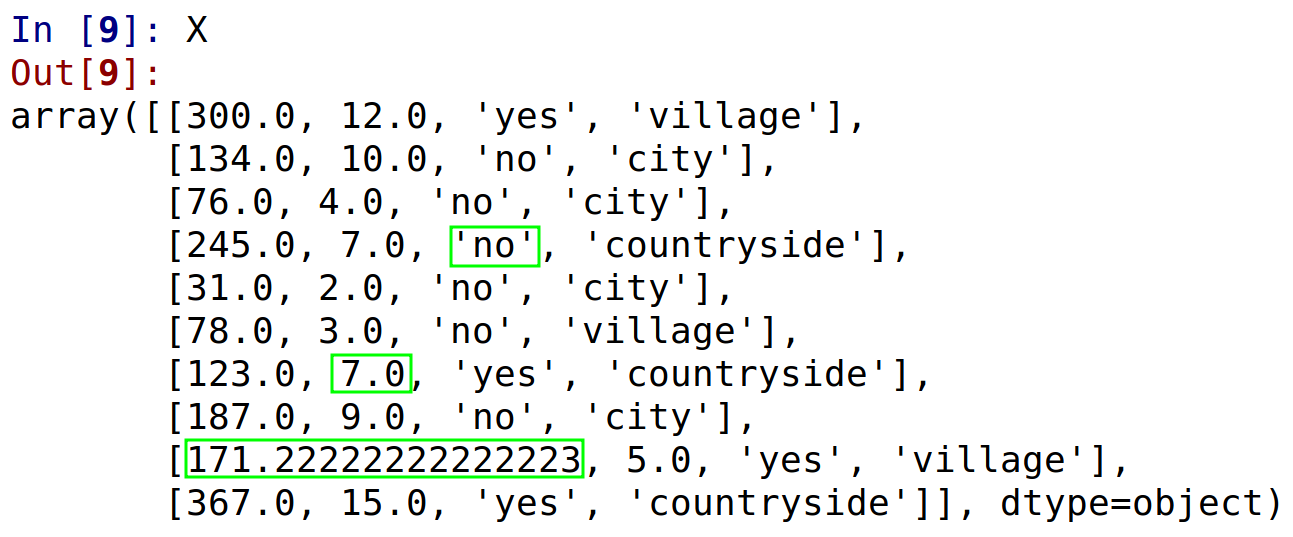
After encoding categorical data:
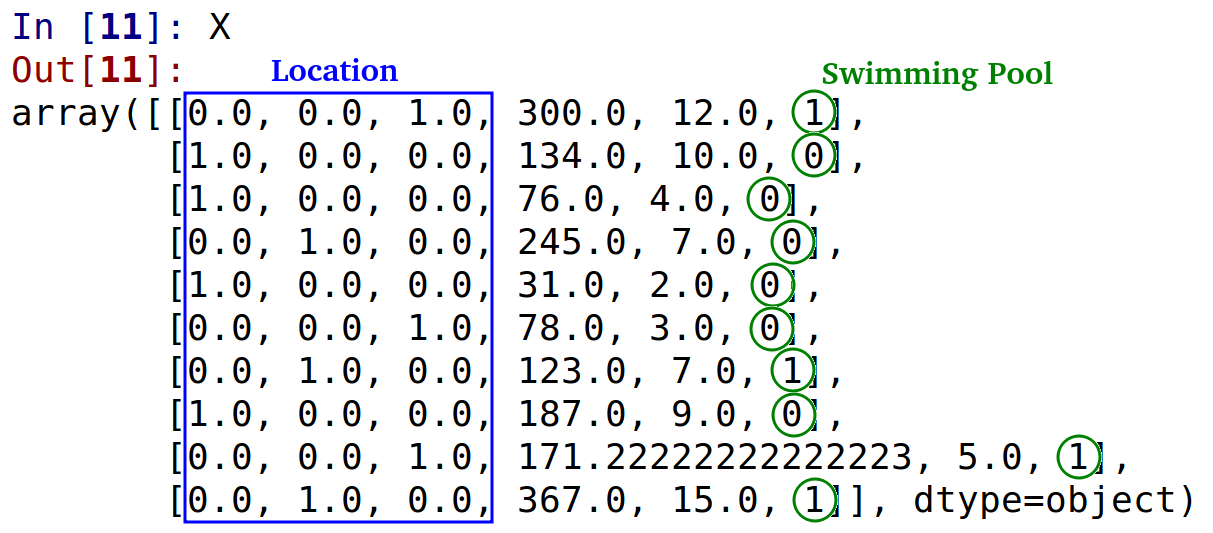
Removing dummy variable:
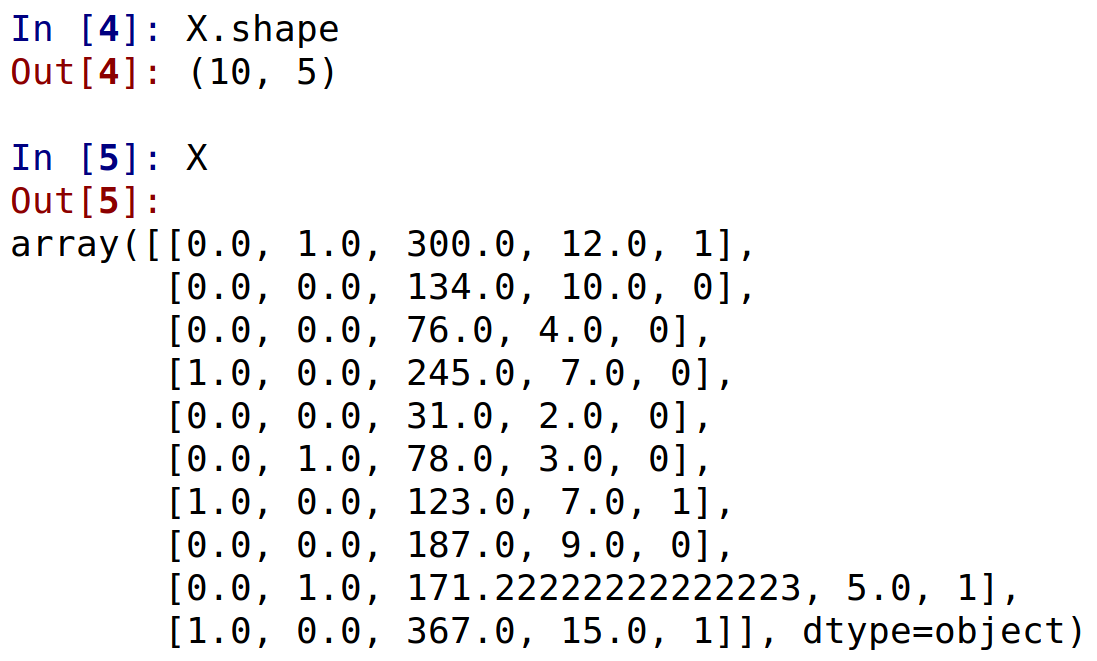
After splitting the dataset in the training set and testing set:
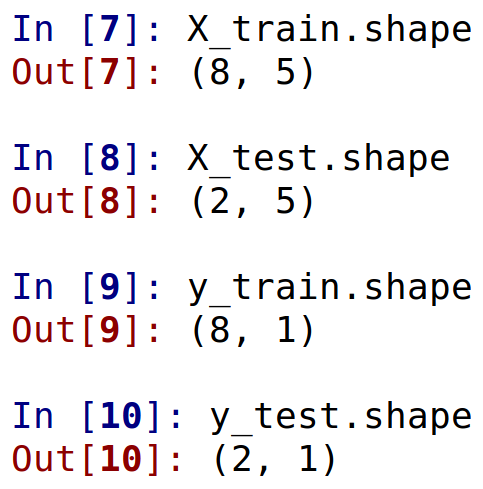
After scaling X and y:
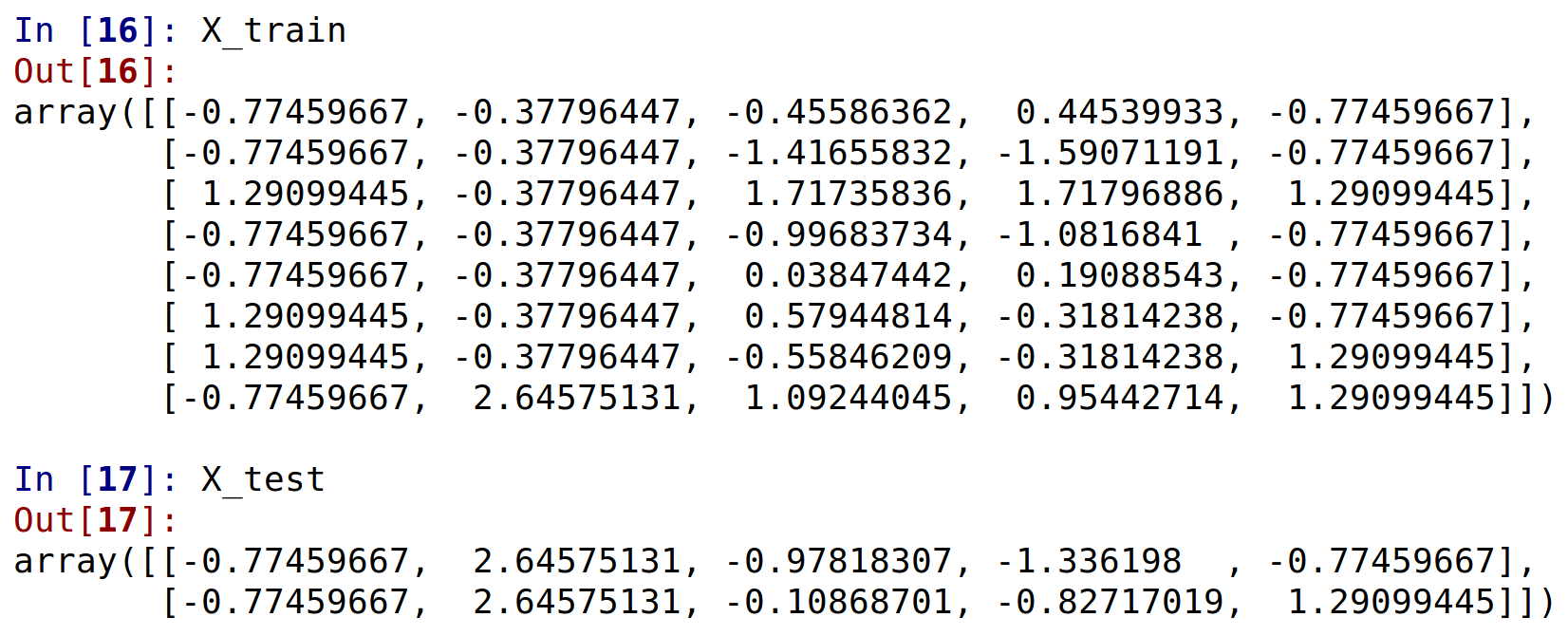
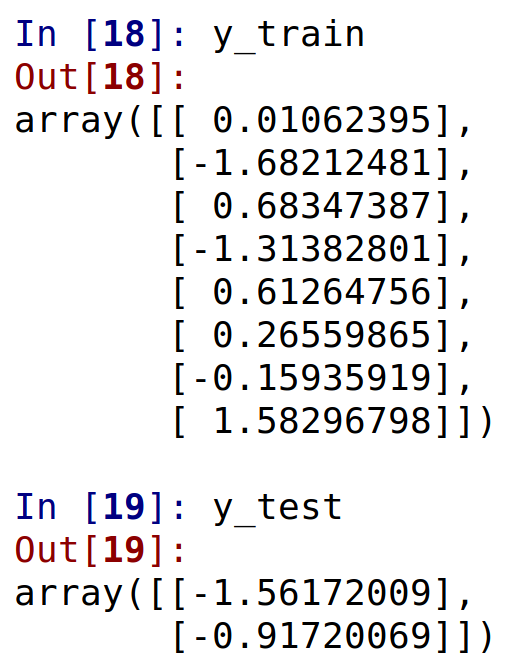